Most Popular Deep learning Frameworks in 2024
Last Updated :
16 May, 2024
Deep learning has revolutionized the field of artificial intelligence, enabling computers to learn from vast amounts of data and perform tasks that were once thought to be exclusive to human intelligence. At the core of these advancements lie powerful frameworks that provide the tools and infrastructure for developing cutting-edge deep learning models. As of 2024, several frameworks have stood out for their efficiency, flexibility, and robustness in facilitating the creation and deployment of neural networks. Here, we delve into the top 10 frameworks that are driving innovation in the realm of deep learning.
In this article, we will explore the most popular deep learning frameworks in 2024, the Capabilities, Features, and Benefits of Each of the Framework
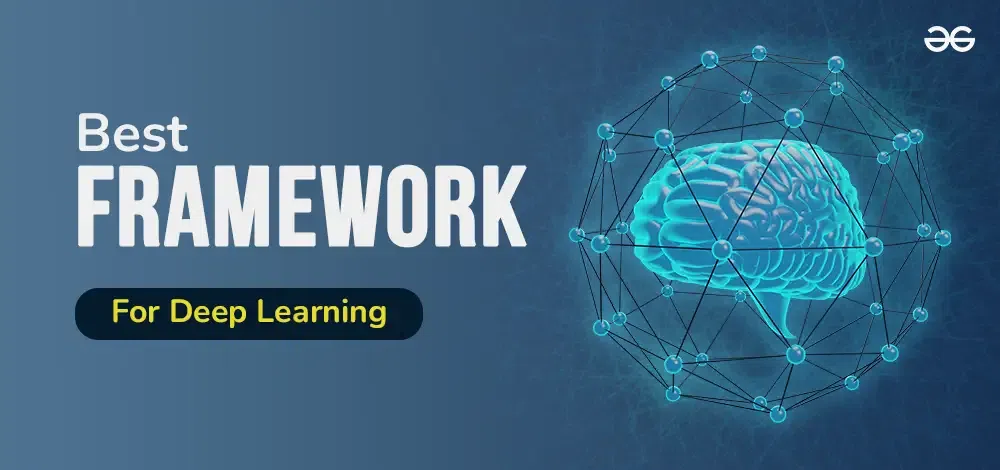
TensorFlow
TensorFlow remains a dominant force in deep learning frameworks. Developed by Google, it boasts a comprehensive ecosystem and supports a wide range of applications. TensorFlow’s flexibility, scalability, and extensive community support make it a top choice for both research and production-level deployments.
- Capabilities: A Toolkit set for numerical computation, machine learning and deep learning which is diverse. Static graph computations and the possibility to deploy models on mobile devices is provided (The object is TensorFlow Lite).
- Benefits: Communities having vast areas and well developed infrastructure, capable of being scaled up and remodel whenever it is required.
- Limitations: It is fair to say such complexity may not be suitable for the beginners often since it requires one to understand the low-level picture. The process of debugging a computation graph can be complicated.
Features of TensorFlow
- TensorBoard: Integrated visualization tool for model graphs, training curves, and histograms of various metrics.
- TensorFlow Hub: A repository for reusable machine learning models and components.
- TensorFlow Lite: Framework for deploying machine learning models on mobile and IoT devices.
You can refer to this article – Introduction to TensorFlow
PyTorch
PyTorch, maintained by Facebook’s AI Research lab (FAIR), has gained immense popularity due to its simplicity, flexibility, and dynamic computation graph. Its intuitive interface and Pythonic syntax make it an ideal choice for researchers and developers, allowing for seamless experimentation and rapid prototyping.
- Capabilities: Flexible neural networks open up the door for fast implementation of the various prototypes. It is cleanly integrated with other commonly used scientific computing libraries of python such as (NumPy, SciPy).
- Benefits: It is accessible for research and development activities thanks to its agile nature and robust debugging capabilities Active research community.
- Limitations: Commonly used when developing dynamic web apps hence may not be as efficient as static frameworks in large deployments.
Features of PyTorch
- Dynamic Computation Graphs: Allows defining and modifying computation graphs on-the-fly during runtime.
- TorchScript: Facilitates exporting PyTorch models to run independently in production environments.
- Support for CUDA: Provides GPU acceleration for neural network computations.
You can refer to this article – What is PyTorch ?
Keras
Keras, now an integral part of TensorFlow, provides a high-level neural networks API that emphasizes ease of use and modularity. It allows for quick iteration and prototyping, making it an excellent choice for beginners while also offering advanced features for building complex models.
- Capabilities: The API at the disposition of the user is related to the creation of deep learning models, which he/she can implement on the frameworks such as TensorFlow, PyTorch, or MXNet. This API emphasizes the ease of use and quick exploration.
- Benefits: This course is suitable for beginners. Ensures code portability across various deep learning framework through machine learning and deep learning tools.
- Limitations: Doesn’t support some of the better features and customization options provided in lower level frameworks.
Features of Keras
- Ease of Use: Offers a simple and intuitive interface for building neural networks.
- Model Reusability: Enables sharing, reusing, and modifying pre-trained models easily.
- Multiple Backend Support: Can seamlessly run on top of TensorFlow, Theano, or Microsoft Cognitive Toolkit (CNTK).
MXNet
Backed by Apache Software Foundation, MXNet offers scalability and efficiency, particularly in distributed computing. Its hybrid frontend allows users to switch between imperative and symbolic programming paradigms, catering to diverse use cases across industries.
- Capabilities: Highly scalable and proficient code structure with the capability to work with more than one language (Python, R, Julia, etc. ). It enables distributed training and model deployment processes.
- Benefits: It is the go-to framework for fast-paced AI tasks handling millions of data that come from various hardware set-ups. The applicability for systems like the mobile and embedded devices.
- Limitations: And when compare to TensorFlow or PyTorch, the community might be smaller and the resources might be lack.
Features of MXNet
- Hybrid Frontend: Allows combining imperative and symbolic programming paradigms for increased flexibility.
- Scalability: Supports distributed computing, making it suitable for large-scale deployments.
- Compatibility with Various Languages: Provides APIs for Python, Scala, and R.
Caffe
Caffe’s strength lies in its speed and efficiency, making it a preferred choice for projects where inference speed is crucial. Although its declarative architecture may seem less flexible than newer frameworks, its simplicity and performance are advantageous for specific tasks. In Caffe’s Python interface, while the primary focus is on defining and training neural networks, the library does offer functionalities for tasks other than neural network creation and training. Caffe’s Python interface allows access to various features such as pre-processing, feature extraction, and network manipulation.
- Capabilities: Consequently, its first motivation is to employ deep learning and convolutional neural networks (CNNs). Cloud computing delivers superior performance as well as its simple requirements.
- Benefits: Modern framework for neural networks with CNNs being focused on performance speed and efficiency. With deployable models becoming popular, it is easy to get started with AI.
- Limitations: Limited in flexibility, they become less adaptable for sophisticated CNN models apart from CNNs. It appears that even if the development continued, it would likely cease in the past years.
Features of Caffe
- Speed and Efficiency: Known for its fast inference times and efficient memory usage.
- Model Zoo: Offers a collection of pre-trained models available for various tasks.
- Python Interface: Provides a Python interface for building, training, and deploying models.
Here are a few examples of tasks you can perform using Caffe’s Python interface beyond neural network construction:
- Feature Extraction: Caffe allows extracting features from intermediate layers of a pre-trained neural network. This can be useful for transfer learning or obtaining representations from specific layers.
- Fine-tuning: You can fine-tune an existing pre-trained model by loading it and adjusting specific layers’ weights based on your new dataset.
- Image Pre-processing: Caffe offers utilities for image pre-processing that can be used to prepare data for training or inference.
Theano
While no longer actively developed, Theano pioneered many concepts in deep learning and symbolic mathematics. Though its popularity has waned, its legacy lives on in the design of subsequent frameworks, showcasing its influence on the evolution of deep learning tools.
- Capabilities: Conventionally known for shallow machine learning activities, TensorFlow changes the game by using the deep learning technique and clean mathematical syntax.
- Benefits: Through this design, created the basis for today’s frameworks. Social Media: Reinforce the understanding of deep learning principles by offering a good practice.
- Limitations: But the growth rate was almost zero (february for the last period). Not recommended for the projects implementation as there no active maintenance works.
Features of Theano
- Symbolic Mathematics: Allows symbolic expression and optimization, facilitating efficient computation.
- GPU Support: Provides support for GPU acceleration.
- Extensive Documentation: Comprehensive documentation and tutorials for users.
You can refer to this article – Theano in Python
Chainer
Chainer’s defining feature is its “define-by-run” approach, providing dynamic neural networks through imperative coding. Although not as widely adopted as some other frameworks, its flexibility and ability to construct models on-the-fly attract users seeking a different paradigm for deep learning.
- Capabilities: The framework can be adapted to create different structures while keeping an object-oriented design. Grants our possibility to do automatic differentiation and improve the system with powerful customization tools.
- Benefits: More based on research because of its flexibility that makes it modifyable by the user.
- Limitations: Communities and resource pools that are smaller and more popular in contrast from other frameworks. Might be more difficult to casually create a game.
Features of Chainer
- Define-by-Run Approach: Offers flexibility in defining neural networks on-the-fly.
- Dynamic Computational Graphs: Supports dynamic graph creation and modification during runtime.
- Built-in Functionality for Reinforcement Learning: Includes tools and functionalities for RL research.
You can refer to this article – How to Install Chainer Python Library on Windows?
Deeplearning4j (DL4J)
DL4J is a Java-based framework designed for scalability and compatibility with JVM languages. Its focus on enterprise applications, distributed computing, and integration with Hadoop and Spark makes it a suitable choice for industries relying on Java-based ecosystems.
- Capabilities: The framework is written in Java; its distributed deep learning is leveraged with Apache Spark. Thick harmful biofilms are formed by some specific types of bacteria, as well as various external contaminants, such as erosion, structural leakage, and dust, and thus they could function like mini-bioreactors for removing contaminations in the local environment.
- Benefits: Java is an ideal platform as it provides an environment for the usage of deep learning models or integration with the Big Data pipelines.
- Limitations: Mostly on Java’s development, this is the main focus. It may not be the best candidate for people, modestly versed in Python, and they will be way better, if their language is CPP
Features of DL4J
- Integration with JVM: Suitable for Java-based applications and integrates well with JVM languages.
- Support for Parallel Training: Enables training models in a distributed manner.
- Compatibility with Hadoop and Spark: Integrates with big data processing frameworks.
Gluon
Gluon, a deep learning interface by AWS and Microsoft, offers an abstraction that combines the ease of use of high-level APIs with the flexibility of low-level APIs. Its hybrid nature simplifies model building while allowing for fine-grained control when necessary.
- Capabilities: Ease of use and flexibility with top-level API Embedded deep learning by constructing MXNet framework. Combines the symbolic and imperative methodologies.
- Benefits: Effective and scalable like MXNet has bigger API comparison to the Keras that is more user-friendly.
- Limitations: As MNIST is conveniently built on MXNet the community might be smaller and less resources compared to TensorFlow and PyTorch.
Features of Gluon
- Hybrid API: Provides a high-level interface for quick model building and a low-level API for fine-grained control.
- Flexibility: Allows defining neural network architectures as code blocks for improved modularity.
- Integration with Apache MXNet: Built on top of MXNet, combining MXNet’s scalability with a user-friendly interface.
TensorFlow.js
As the only JavaScript library on this list, TensorFlow.js enables training and deploying models in the browser or Node.js environment. Its accessibility and seamless integration with web technologies open up possibilities for running deep learning models directly in web applications.
- Capabilities: Enables training and/or running deep learning models in web browsers or Node without transferring created data to a cloud server or another computational environment. js environments using JavaScript.
- Benefits: This allows one to deploy modern deep learning models being used for these products in web applications without depending on server-side processing.
- Limitations: In such case though, a model’s complexity and performance on CPU or GPU bases limit how sophisticated it is.
Features of TensorFlow.js
- Runs in Browser: Allows training and running models directly in web browsers.
- WebGL Acceleration: Utilizes GPU acceleration through WebGL for improved performance.
- Supports Node.js: Enables server-side JavaScript execution for machine learning tasks.
You can refer to this article – Tensorflow.js Introduction
Conclusion
In conclusion, the landscape of deep learning frameworks continues to evolve, offering a diverse set of tools and approaches to cater to various needs in the field. While TensorFlow and PyTorch maintain their dominance, newer frameworks like Gluon and TensorFlow.js signify the continuous innovation in making deep learning more accessible and versatile across different domains and platforms. The choice of framework often depends on specific project requirements, emphasizing the importance of understanding the strengths and trade-offs offered by each framework to harness the full potential of deep learning in 2024.
Share your thoughts in the comments
Please Login to comment...