What is Data Lineage? – Defintion, Tools and Examples
Last Updated :
10 May, 2024
With data increasingly powering key business decisions, understanding data lineage has become crucial for trust and transparency. Data lineage isn’t just a fancy term; it’s a powerful tool that acts like a detailed roadmap for a specific piece of data. It tracks the data’s entire journey, from its very origin to its final destination and every transformation it undergoes along the way.
This article provides a comprehensive overview of what is data lineage, its use cases, benefits, best practices, and tools to establish end-to-end visibility into your data supply chain.
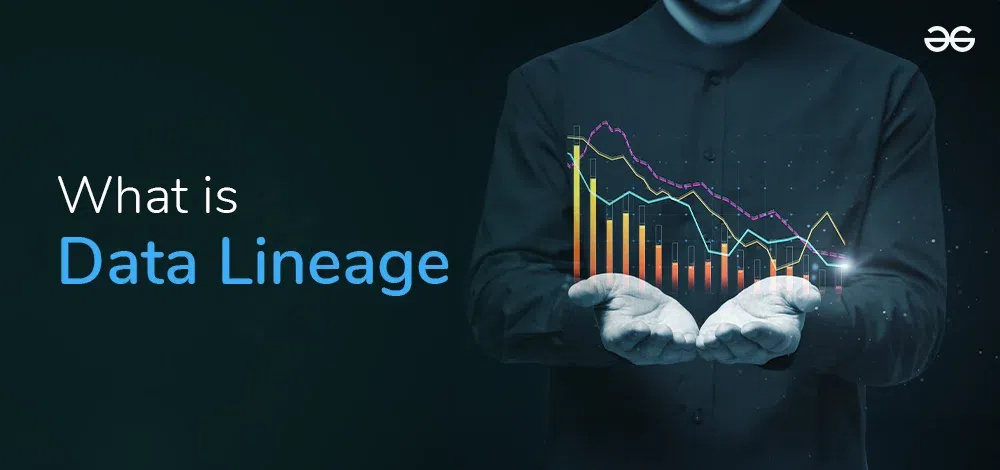
What is Data Lineage?
Data lineage refers to maintaining a record of the origin, movement, and processing history of data from its birth to usage. It provides a complete audit trail tracing data as it is transformed, combined, and propagated across systems.
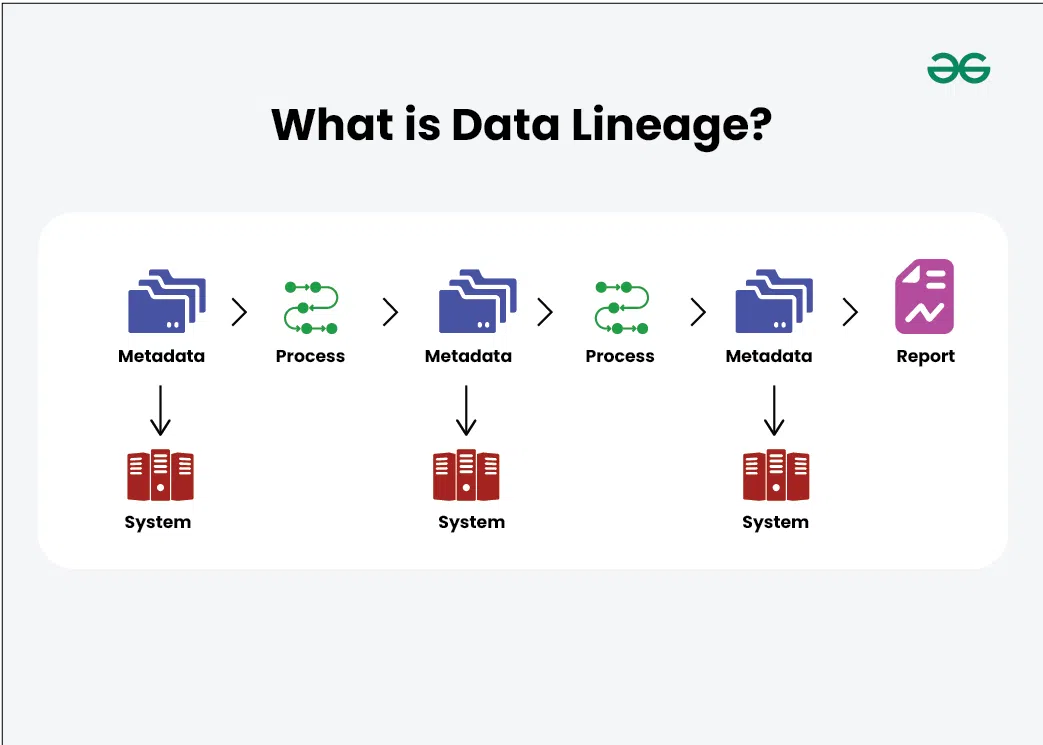
What is Data Lineage
Data lineage captures essential metadata like:
- Where the data originated from
- How it flows between databases, processes, analytics
- Who or what algorithms have accessed/modified it
- The sequence of operations performed on the data
- Think of data lineage as a breadcrumb trail documenting the full lifecycle journey of data as it gets created, consumed, enriched, archived, or deleted.
Why is Data Lineage Important?
Now we know what is Data Lineage, Looks explore it’s importance. Here are some key reasons why data lineage delivers immense business value:
- Trust: By providing data source transparency, lineage enables stakeholders to trust insights and make decisions confidently.
- Auditability: Lineage forms the basis for compliance, governance, and regulatory reporting by auditing data trails.
- Impact analysis: Allows tracing back from outputs to the specific roots and transformations that contributed to them.
- Error diagnosis : When issues arise, data lineage helps pinpoint the point of failure by replaying the sequence.
- Recoverability : Data provenance aids disaster recovery, backup, and business continuity efforts.
- Reproducibility : Retracing the steps makes it possible to reproduce experiments and retrain models.
Data lineage delivers confidence, integrity, and analytical rigor throughout the data lifecycle.
How Data Lineage Works?
Now we know what is Data Lineage, and why is it important. Now we’ll move onto the next step of learning How does Data Lineage Works?
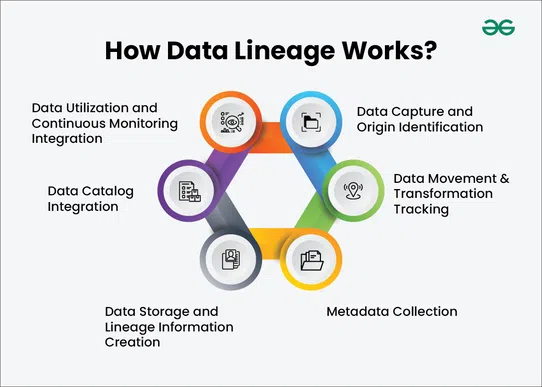
What is Data Lineage? – Working
Data lineage tracks the journey of a specific piece of data, providing a detailed roadmap of its origin, transformations, and final destination. Here’s a breakdown of the typical steps involved:
- Data Capture and Origin Identification: The process begins with identifying the starting point or the resource where the data comes from. This can be a customer’s sign-up form on a webpage, a sensor in a production facility, or any other component that operations into this nature.
- Data Movement and Transformation Tracking: Data is covered by these data lineage tools via process and the system thus the movement. This processing implies the kinds of transformations that may include cleaning, filtering and enrichment (combining it with additional data).
- Metadata Collection: Metadata, which we can define as the “data about data”, performs a very important function in the process of lineage. Data will leave the metadata behind that will be informative about its traits, for example, its format, creation date, and the executed transformations.
- Data Storage and Lineage Information Creation: The center of the repository the smart meter captures these data and sends through the smart grid t move calculations down. By data lineage tools, this data is used to create automatically a lineage map that illustrate and track the data path between data system.
- Data Catalog Integration: In most situations data-lineage is in the data catalogue with the data catalog. The central library of data lineage is accessible to the users, which means that they can find any information about say raw data used to convey a given message.
- Data Utilization and Continuous Monitoring: Business analysts, data scientists, and other stake holders can use the data catalog to investigate the data provenance and this information helps them to understand the uses of the data. This transparency means that they have use of the most accurate, high-quality data possible to conduct in-depth, balanced analysis and decision-making.
It’s important to note that implementing data lineage can involve a variety of tools and techniques. The specific steps involved may vary depending on the organization’s data ecosystem and chosen approach.
Challenges in Tracking Data Lineage
However, establishing robust data lineage poses several challenges:
- Explosion in volume and velocity of big data makes tracking exhaustive metadata difficult.
- Complex interconnected ecosystems of tools, pipelines, and stakeholders increasingly touch data.
- Manual documentation and updating of data flows becomes infeasible at scale.
- Legacy systems lack native mechanisms to capture lineage, necessitating custom integration.
- Frequent changes to architectures, code, and data break recorded mappings.
- Distinguishing transformations vs direct copy relationships requires intelligence.
To address these challenges, two primary technical approaches are employed:
This method relies on manually defined metadata models that encapsulate relationships, technical schemas, owners etc. Tools like data catalogs provide a structured way to manage this metadata. Users can define lineage by explicitly linking data sets and processes. It is Simple to implement with existing tools and flexible custom metadata models. However requieres manual efforts needed for documentation and prone to outdatedness.
2. Automated Lineage Tracing
This technique uses automated agents to dynamically capture granular lineage as data flows through pipelines. Code instrumentation, log parsing, and network sniffing reveal data trajectories across technologies. Changes propagate automatically. Provides complete, up-to-date lineage with minimal manual effort. and agnostic to data architecture and languages but with Increased overhead and complexity and large volumes of usage logs and dependencies to parse.
Hybrid approaches combining some manual metadata definition with automated lineage filling the gaps are common.
Best Practices for Data Lineage
Here are some best practices for implementing data lineage in your organization:
- Standardize Naming Conventions: Enforce consistent naming conventions for data elements throughout your systems. This simplifies tracking data flow and reduces confusion, especially in complex environments.
- Automate Lineage Collection: Leverage automated data lineage tools to capture data transformations and lineage in real-time. Automation minimizes human error and ensures the accuracy of your data lineage records.
- Document Data Sources Meticulously: Maintain detailed documentation of all data sources within your organization. This includes information about the data format, update frequency, and any relevant access controls.
- Define Clear Ownership and Stewardship: Establish clear ownership and stewardship for data assets. This ensures accountability for data quality and facilitates communication regarding data lineage.
- Utilize Visualization Tools: Use data lineage visualization tools to create easy-to-understand diagrams that illustrate the flow of data. These diagrams should be tailored to the audience, with technical details for data architects and high-level overviews for business users.
- Continuously Audit and Update: Regularly review and update your data lineage information to maintain its accuracy as your data landscape evolves. Schedule periodic audits to identify and address any gaps or inconsistencies.
- Focus on Completeness: While aiming for comprehensiveness, avoid getting bogged down in excessive detail. Strive to capture the essential transformations and flow of data relevant to your needs.
Types of Data Lineage Tools are discussed below:
- Data Catalogs (Collibra, Alation): Build an intermediary system that will maintain data definitions and simple lineage information.
- Workflow Managers (Apache Atlas, Azure Purview): Harvest heredity lines by virtue of data pipelines they were in charge of.
- ETL Tools (Informatica, Talend): Provide a lineage function to deal with transformations where that task is done in the environment where the process is executed.
- Lineage Platforms (DataEdna, Monte Carlo): Focus on automating data lineage tracing from source to end device with different services deployments.
- Graph Databases (Neo4j, Spark): Utilize graphs as a data structure to not only store but also visualize data relationships even those containing lineage.
- Lineage Visualization Tools (Linkurious): Present interactive interfaces to get into detail with and comprehend the routes shown on the map.
This categorization helps organizations choose the right tool based on their specific needs and data ecosystem complexity.
Data Lineage Techniques and Examples
Here are some common data lineage techniques:
- Manual Techniques: This involves documenting the data flow through interviews with data engineers, analysts, and other stakeholders. While comprehensive, this method is time-consuming and error-prone.
- Pattern-Based Lineage: This automated approach analyzes data metadata (information about the data itself) to identify patterns and relationships between data points. For instance, similar column names and data values in two tables might suggest a connection.
- Query History Tracking: This technique monitors user queries and reports generated from databases and data warehouses. By understanding these transformations, data lineage can be established.
Here’s an example to illustrate data lineage:
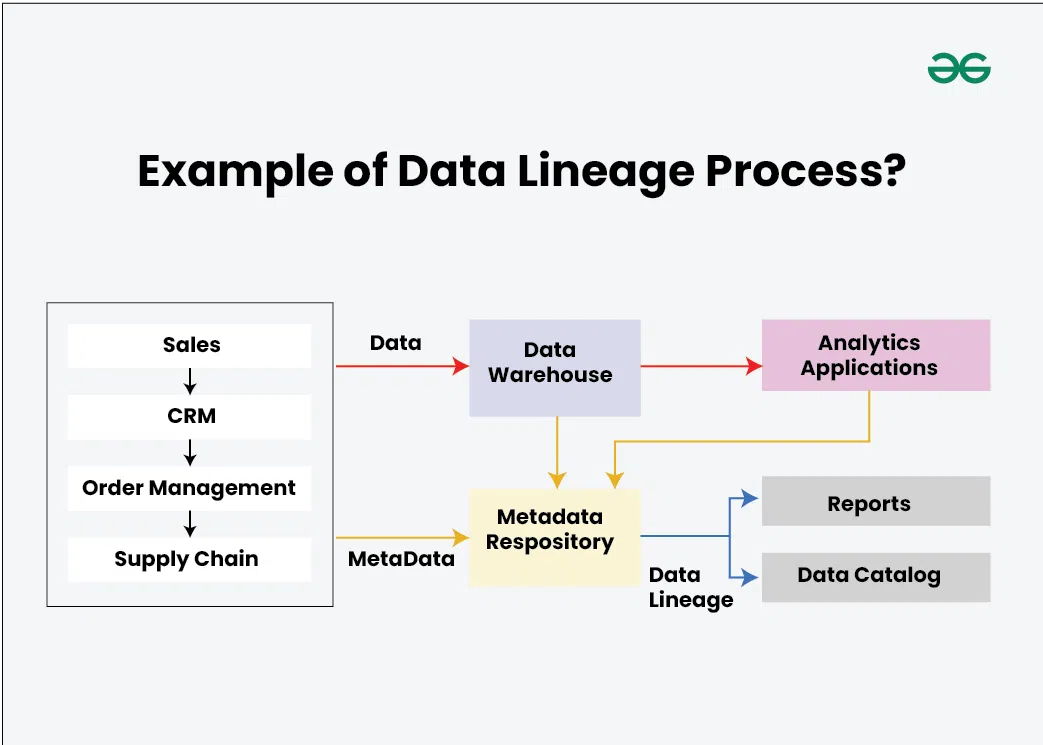
Example of Data Lineage Process
Imagine a customer signs up on a company website, providing their information on a signup form. This data, the origin of the customer record, then travels to a Customer Data Platform (CDP) where it undergoes a transformation. Here, duplicates are removed and the data might be enriched with additional details from other sources like purchase history. Following this, the enriched customer record arrives at the CRM system. Throughout this flow, metadata tracks the data’s journey, including its origin, any transformations, and timestamps. This metadata is then used to create data lineage information stored in a Data Catalog. By utilizing the Data Catalog, business analysts can understand the complete story of the customer data, from its initial capture to its use in reports and dashboards. This transparency ensures they are working with high-quality, reliable data for accurate insights.
Why Businesses Invest in Data Lineage?
Businesses are investing in data lineage for several key reasons:
- Trust and Transparency: Data lineage planning is the perfect auditing trail that is necessary for compliance with a data security regimen (such as GDPR and CCPA). It further enables great data oversight by ensuring that power on the data use remains rooted within the organizations.
- Data Quality and Efficiency: Lineage tool further allows for automated metadata capturing, and therefore the single window of truth concerning data elements and their ownerships is ensured. The ability of automation to do such tasks makes data discovery faster and impact analysis easier even if data sources or systems change.
- Improved Analytics and AI: Through interrogating the lineage of data, analysts discover the origin or provenance of data which helps them identify the data points relevant for their projects. This can be used further to enhance the trust of AI and make sure that the models use high-quality data that can be traced back to the source.
- Faster Debugging: During problem resolution periods, data lineage maps serve as a tool to detect exactly where and how the error or the bias got into the data stream hence speeding up the error localization.
Data Lineage Applications
Some of the Common Application of Data Lineage are discussed below:
- Data Governance – Data lineage provides visibility into the origins and movement of data across systems. This enables improved governance by tracking data from source to destination, highlighting areas of risk or non-compliance.
- Data Quality – By mapping data flows, lineage helps identify root causes of data quality issues such as systemic biases or errors introduced during processing. Data issues can be remediated more quickly.
- Metadata Management – Data lineage maps support metadata management by documenting dependencies, data domains, owners, custodians and processes associated with datasets. This enhances discoverability.
- Impact Analysis – Lineage allows assessing downstream impact of changes to data assets or sources. This is crucial for planning system migrations, compliance with regulations, or when sources are deprecated.
- Auditing – Data lineage provides audit trails for demonstrating compliance, assigning accountability, and investigating issues. Regulators often require auditable lineage.
- Data Discovery – By revealing relationships and origin points, lineage helps analysts find relevant datasets for analysis and gain insights into how metrics are derived.
- Debugging – When debugging pipelines or investigating failures, data lineage maps precisely pinpoint where and how errors were introduced into the data flow.
- Model Governance – ML models depend on specific data inputs and processes. Lineage illustrates model governance and compliance by mapping model data supply chains.
Data Lineage vs. Data Provenance vs. Data Governance
Data lineage, data provenance, and data governance are distinct yet interconnected concepts.
Aspect
|
Data Lineage
|
Data Provenance
|
Data Governance
|
Focus
|
Traces the flow of data through its lifecycle.
|
Emphasizes the origin, creation, and history of data.
|
Encompasses the overall management and control of data.
|
Purpose
|
Understands the path of data, from source to destination.
|
Highlights the lineage of data creation and changes.
|
Manages and ensures the quality, integrity, and compliance of data.
|
Components Tracked
|
Sources, transformations, and destinations of data.
|
Origin, changes, and movement of data over time.
|
Policies, standards, processes, and overall data assets.
|
Key Benefits
|
Enhances data quality, supports compliance, and aids troubleshooting.
|
Improves transparency, supports forensic analysis, and aids in error detection.
|
Ensures data quality, compliance, and overall effective management.
|
Use Cases
|
Troubleshooting, compliance, data quality management.
|
Forensic analysis, error detection, and historical context.
|
Compliance assurance, risk management, and overall data control.
|
Automation
|
Can be automated to capture and update data lineage.
|
Automation can assist in tracking data changes over time.
|
Automation in enforcing data policies and standards.
|
Visualization
|
Graphical representation of data flow and transformations.
|
Visual representation of the historical evolution of data.
|
Policies and standards are often documented and visualized.
|
Examples
|
Showing how customer data moves from CRM to analytics.
|
Tracking changes in a dataset over weeks or months.
|
Implementing policies for data access, usage, and retention.
|
FAQs on Data Lineage
Q. What are some key benefits of data lineage?
Data lineage enhances trust, reproducibility, auditability, impact analysis, diagnostics, and recovery – providing end-to-end transparency.
Q. What are some data lineage best practices?
Integrate lineage into processes, enrich metadata, sustain mappings against changes, validate periodically, automate collection, and surface through catalogs and visualizations.
Options include data catalogs, ETL platforms, workflow managers, graph databases, data lineage products, and visualization dashboards.
Q. How is data lineage different from data profiling?
Data profiling analyzes properties of a dataset while lineage tracks the origins and processing steps across systems. The two are complementary.
Q. Is data lineage required for compliance?
Yes, regulations like GDPR have explicit requirements for data audit trails that lineage provides. It is vital for compliance.
Share your thoughts in the comments
Please Login to comment...